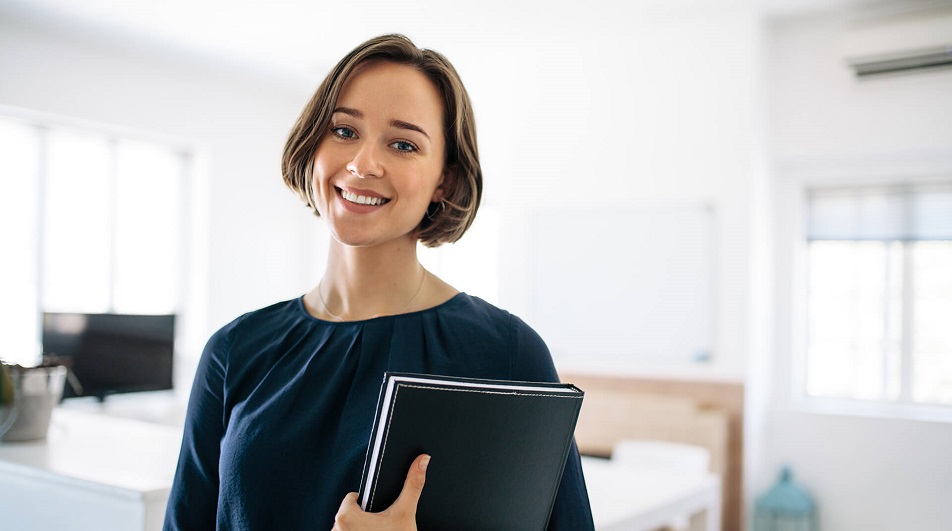
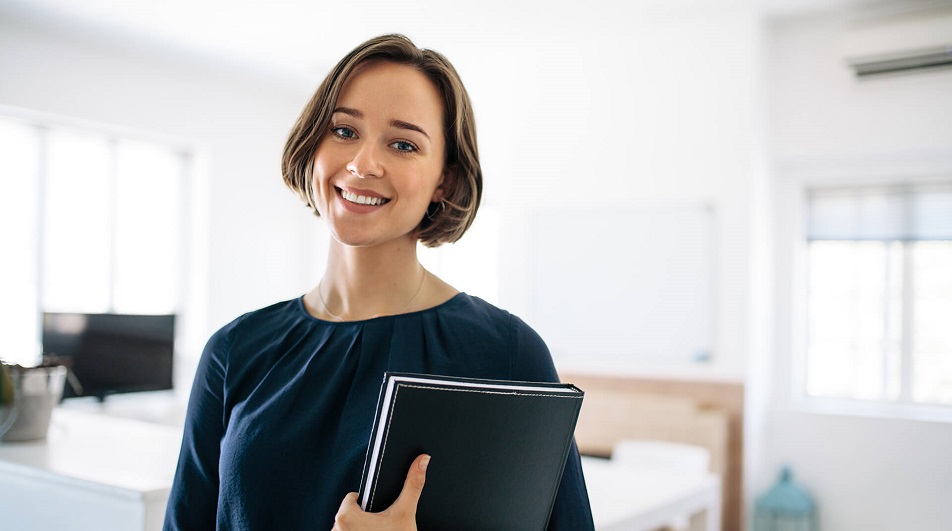
- April 17, 2023
- colossusanalyticsllc@gmail.com
- 0
If you are planning to grow and expand your business, you need to be making more data-driven insightful business decisions. And to make such informed decisions we need to rely on credible, accurate and insightful predictive analytic methods. One such method that is used popularly is using machine learning to boost predictive analytics capabilities.
Today we will be covering how machine learning can be used for predictive analysis. But before that lets understand what Predictive Analysis and Machine Learning are and why you should care.
What is Predictive Analysis?
As the name suggests predictive analysis is the procedure of analyzing data, statistical algorithms as well as machine learning algorithms for identifying the possible future outcomes based on current and historical data. This can help companies such as yours to identify new opportunities ahead of time, anticipate customer needs and reduce risks by making calculated decisions.
What are the types of Predictive Analysis?
Almost all businesses have chunks of business data, both structured and unstructured that can be utilized for predictive analysis and fulfilling their business objectives. However, there is more than one way of data analysis. They are –
Type of Data Analysis | Description |
Descriptive Analysis | Such analysis works on summarizing historical data for gaining insights on past events and trends. Such type of analysis doesn’t make predictions about the future. |
Predictive Analysis | Here we use statistical algorithms and machine learning for analyzing historical data to make predictions about future events and possibilities. |
Prescriptive Analysis | Here we use data, algorithms and machine learning to make recommendations that would help us achieve a desired outcome. |
What is Machine Learning?
Machine Learning can be called a subset of Artificial Intelligence which enables systems to learn and improve their outputs from experience without having to be explicitly programmed. Machine learning works on statistical algorithms and computer models for analyzing data, identifying patterns, the relationships between different patterns and drawing inferences and insights that can help users make informed predictions and subsequently informed decisions.
Types of Machine Learning Methods
There are many ways to use machine learning for achieving different results. Three of the most widely used machine learning methods you should know about are –
Type of Machine Learning Methods | Description |
Supervised Learning | The machine learning algorithm gets trained on a labeled dataset with desired output already determined. The algorithm learns how to identify patterns and relationships to make predictions on new data. |
Unsupervised Learning | Here the desired output is unknown and the goal of unsupervised learning is to discover the existing data structure and group similar data points together. This can be further used for data visualization requirements. |
Reinforcement Learning | Here the machine learning algorithm learns how to make decisions by interacting with a given environment and receiving feedback as rewards or punishments for correct and incorrect predictions respectively. |
Machine Learning Predictive Analysis Use Cases
There are many industries that can leverage machine learning with predictive analysis to derive some useful strategic insights and help businesses make more strategic decisions to enhance their operations, improve profitability and gain many more advantages. Here are some industries we at Colossus Analytics have worked with for implementing machine learning predictive analysis.
ML with Predictive Analytics in Ecommerce
Performing predictive analytics with machine learning capabilities for ecommerce owners can be very helpful in identifying and understanding consumer behavior and preferences. Machine learning can be set to read and analyze events such as click-through-rates of specified products, understanding patterns between two different ecommerce products and other such insights which can help ecommerce merchants better place their recommended products, provide discounts to drive customer conversion and much more.
ML with Predictive Analysis in Sales and Marketing
ML can be used in the Sales and Marketing industry for identifying and converting prospect clients based on the attributes that match your existing customer base. It can also be used for identifying and prioritizing known prospects, improving customer conversation rates, and streamlining marketing and sales operations with data driven insights instead of shooting aimlessly.
ML with Predictive Analysis in Health Diagnosis
One of the most important aspects of improving the chances of one’s livelihood and overall health conditions is being able to provide more accurate health diagnosis at the right time. Predictive analysis has a huge potential for solving this issue as healthcare professionals have access to previous patient medical history and reports. Using these information points, we can create accurate, reliable and highly performant algorithms to study and identify patient symptoms better.
How Machine Learning is used for Predictive Analysis?
Machine Learning in predictive analysis can be used for identifying patterns, relationships and strategic insights that can be used for predicting future outcomes. By feeding historical data into machine learning solutions, its algorithms can identify trends and patterns that can be interpreted by business owners and marketing professionals to make accurate predictions. You could also use machine learning to identify the most important variable that contributes to your overall success or find the variable that’s mostly causing performance bottlenecks and challenges.
Process of using Machine Learning in Predictive Analysis
If you wish to implement machine learning in your predictive analysis technique, this is the general process for achieving it –
Step 1 – Data Collection
You will first work on collecting data and preparing it for machine learning. This means you need to identify the relevant variables and have sufficient data over the time under these variables.
Step 2 – Data Preprocessing
After collecting the data, it gets further refined and preprocessed for removing missing values, irrelevant information or outliers. It is important to ensure that the data is complete and accurate for building proficient machine learning models.
Step 3 – Feature Selection
This is a crucial step where we need to select the most relevant features or variables for the predictive analysis. The feature selection should be based on finding features with strongest correlation with target variable or outcome that we want to predict.
Step 4 – Model Selection
Next, we need to select the most appropriate machine learning model. This would again depend on what we want to achieve with this predictive analysis process and factors like size and complexity of the dataset, desired accuracy levels and more.
Step 5 – Model Training
After that, we start training the selected machine learning model on historical data for identifying patterns, relationships and more that help make future predictions.
Step 6 – Model Evaluation
All the training needs to be evaluated to see if the machine learning model for predictive analysis is accurate and we can make some minor refinements as and where needed. After that it is all set to be deployed in real-world settings to make predictions about future events.
Final Words
Machine Learning paired with predictive analysis can help any business take more strategic decisions and reduce unnecessary expenditure in performing trial and error tests which can waste a lot of time and cost a fortune. If you are looking to optimize your business with ML, consult with our dedicated machine learning consulting team today.